Shifts
The Shifts Project is an international collaboration of academic and industrial researchers dedicated to studying distributional shift — one of the biggest challenges in applying machine learning to high-stakes real-world tasks.
The goal of the project is to raise awareness of distributional shift, collect datasets and benchmarks, facilitate cross-disciplinary research, and organize challenges and workshops.
About
What is distributional shift?
Distributional shift is the mismatch between training and deployment data. Generalizing to ‘shifted’ data is challenging for ML models. Typically, the performance of ML modes will drop as the degree of shift increases. For example, both human and AI drivers trained to drive on the right side of the road can struggle in countries with left-sided driving. Distributional shift is ubiquitous in machine learning and is especially important to be aware of in safety-critical applications.
What are the obstacles?
Until recently, most work on improving robustness to shift and predictive uncertainty estimation has been conducted on small-scale image classification datasets which contain synthetic or unrealistic forms of distributional shift. Conclusions drawn from these datasets rarely carry over to industrial-scale ML applications. The lack of large, diverse and industrially sourced datasets with examples of ‘real’ distributional shifts makes it difficult to validate new approaches and reach conclusions that are broadly applicable to real-life applications.
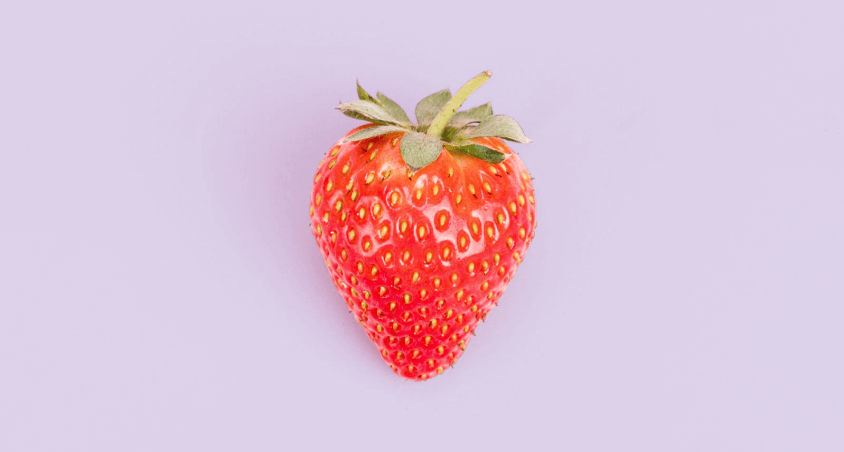
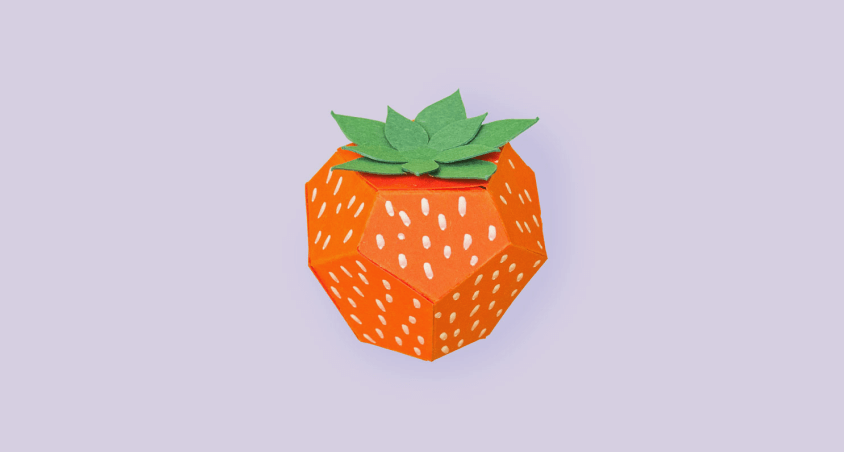
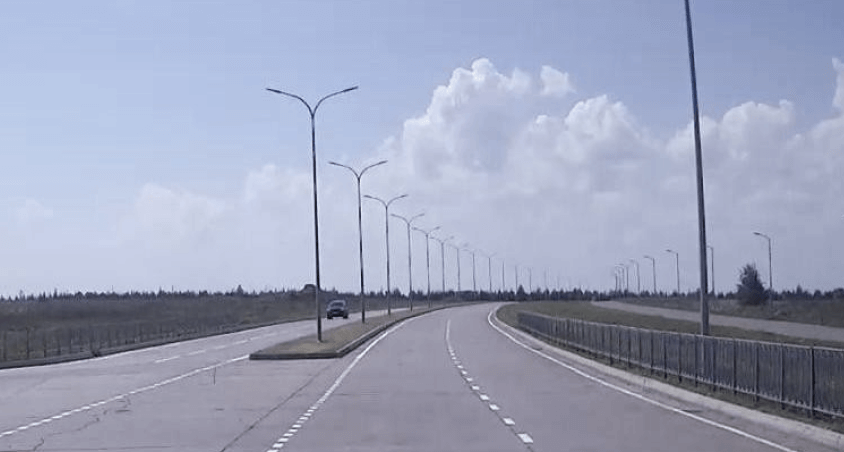
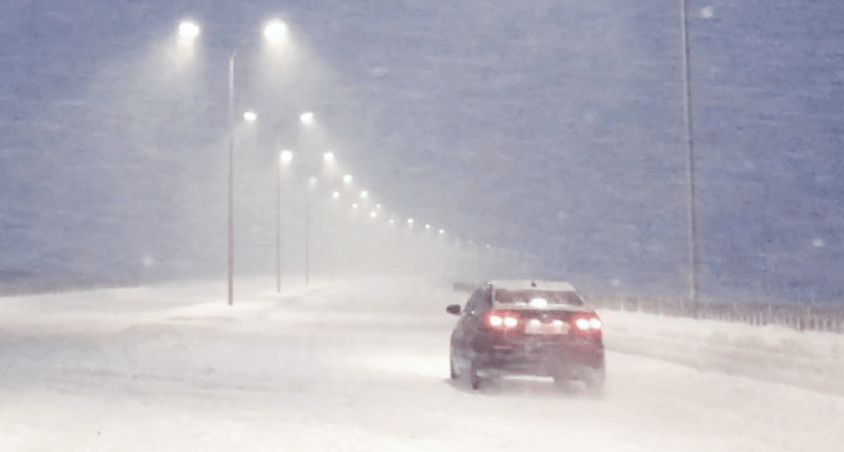
What can we do about it?
Ideally, machine learning models should generalize well under a range of distributional shifts. If they fail to generalize, these models should indicate this using uncertainty estimates, which enables us to take actions that improve the safety and reliability of the ML system. Techniques that induce invariant or equivariant representations, prevent feature collapse and allow learning more information from data will improve generalization. Techniques which yield quantities sensitive to the degree of distributional shift will yield better uncertainty estimates.
Our goal
The goal of the Shifts Project is to build a cross-disciplinary international community, bringing together core-ML and applied ML researchers. Through this we aim to collect new datasets sourced from industry that feature ‘in-the-wild’ distributional shift and make them freely available. This will enable better research in the area with outcomes that can be directly applied in-production. The Shifts Project will be organizing events, challenges and workshops to popularize these new datasets and raise awareness of the problem of distributional shift among the general ML community.
News & Events
See more news-
Shifts Challenge 2022 begins!
The Shifts Project is Happy to announce the launch of the Shifts Challenge 2022! This will be the second iteration of the Shifts Challenge, which successfully debuted last year at NeurIPS 2021.
Published: 15 September 2022 -
The “Shifts” project launches!
We are pleased to announce the launch of the Shifts Project — an international collaboration of academic and industrial researchers dedicated to studying distributional shift in real-world applications.
Published: 15 September 2022
For general questions about Shifts, write to info@shifts.ai or create an issue in our GitHub repository.